MarTech Landscape: What is predictive advertising?
The combination of big data and machine learning enables professionals to efficiently expand their targeting efforts.
Predictive advertising is yet another area of marketing that is evolving rapidly thanks to the massive strides in the strain of artificial intelligence called machine learning and wide access to large sets of digital data.
In this installment of our MarTech Landscape series, we look at how predictive advertising works and how it’s commonly applied.
What makes it predictive?
Predictive advertising is a subset of predictive analytics, also covered in our MarTech Landscape series. Predictive analytics uses machine learning to predict future outcomes based on behavioral patterns seen in historical data. Those predictions can be used for any number of purposes: understanding who is likely to pay off a loan, prioritizing leads most likely to close and so on. In this case, the predictions are used in ad targeting and media buying.
Machine learning is an application of artificial intelligence (AI). Machines are given access to large sets of data and coded to continually learn from the data in order to predict future actions with a high degree of certainty. With predictive analytics powering ad tech, campaigns can target audience segments based on a huge number of behavioral signals, ads can be personalized to be more relevant in the context of the user, and bids can be optimized based on user data — all faster and with higher success rates than humans can manually.
Predictive approaches can anticipate which user might respond to an ad based on a number of different attributes. As with any machine learning-based system, predictive advertising requires large amounts of data for the models to keep training and learning. The more data a system can pool across users and/or advertisers, the faster and better the models can train and learn.
How is predictive advertising changing advertising?
There are several ways in which predictive advertising is being applied to traditional digital advertising tactics, including campaign optimization, media mix modeling, media buying and ad serving. Instead of relying on rule settings, machine learning models are applied to make decisions.
Prospecting or Pretargeting — Predictive advertising can help marketers cast a wider net beyond people or businesses they may have identified otherwise using limited data or — shudder — intuition. Predictive advertising can be used to identify and target new prospects with ads based on what is known about a company’s existing customers or visitors. Predictive advertising platforms can suck in structured data from numerous sources across multiple channels and marketing platforms to develop customer profiles. The systems can then identify and target users who fit those profiles with digital ads.
Lookalike and similar audience targeting offered by Facebook, Google, Twitter and other platforms are examples of predictive advertising applications. Lookalike and similar audiences are built from source audiences that can come from the advertiser, a data partner or the platforms themselves. The models identify other users that look like – have similar attributes and behaviors as — users in the seed lists.
Similarly, in-market audience targeting identifies users as they are deep in the research and buying process — way down the funnel — and enables advertisers to reach them with related messages in near-real time.
Private companies in this arena include AdRoll, which launched its prospecting engine in 2015 to pool advertisers’ first-party customer intent data to inform targeting. EverString has developed a predictive advertising platform to identify and target their B2B customers’ prospects with ads.
Retargeting— In typical retargeting, marketers can segment audiences based on the actions they took on their sites — visited product pages, abandoned a cart, made a purchase, downloaded a white paper and so forth. Predictive retargeting can add layers of data to map out customer intent signals beyond actions taken on their sites to provide much richer targeting capabilities.
Criteo Predictive Search employs machine learning to automate Google Shopping campaigns, including retargeting to “re-engage high-value users via behavioral targeting technology that programmatically sets bids based on each user’s propensity to make a purchase.”
Ad Mediation — Tapjoy applies predictive analytics to optimize ad serving for app publishers. To ensure app publishers don’t miss out on potential in-app purchase revenue, Tapjoy uses predictive systems to identify users who are likely to make in-app purchases. It serves marketing messages to those users, and ads to the larger majority the system predicts won’t make an in-app purchase.
Campaign optimization — Predictive bidding algorithms can adjust in real time based on what the system knows about the session user. For example, Google and Bing Ads both offer conversion- and click-based bid strategies in which bids are adjusted based on the users’s predicted propensity to click or convert.
Performance Horizon has a campaign performance prediction tool baked into its platform for publishers to manage affiliate and partner programs. Using historical data from at least 30 days of a campaign, the platform selects one of five different prediction models as the most appropriate for each client. It predicts sales and commissions based on a current campaign and can identify anomalies.
What does predictive advertising look like?
Here’s a visual from Quantcast illustrating how the company has adapted its systems to enable real-time predictive bidding.
Tens of thousands of models are available in real time from the “Model Store.” The “Real Time Featurizer” collects real-time behavioral data and translates the data into a format the machines can read. Quantcast’s “Keebler” is like a cookie data warehouse from which all of the anonymous user IDs are served — fast.
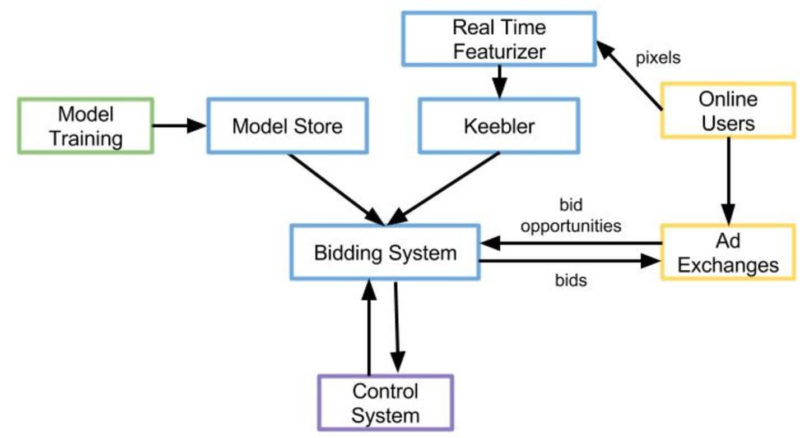
Having immediate access to models and behavioral data enables the system to identify relevant audiences and make real-time bidding decisions based on a user’s predicted interest in a particular product or service.
With the evolution of machine learning’s integration into ad tech systems, it’s now inconceivable to think of a day when predictive advertising is just called advertising.
Marketing attribution and predictive analytics: A snapshot
What it is. Marketing attribution and predictive analytics platforms are software that employ sophisticated statistical modeling and machine learning to evaluate the impact of each marketing touch a buyer encounters along a purchase journey across all channels, with the goal of helping marketers allocate future spending. Platforms with predictive analytics capabilities also use data, statistical algorithms and machine learning to predict future outcomes based on historical data and scenario building.
Why it’s hot today. Many marketers know roughly half their media spend is wasted, but few are aware of which half that is. And with tight budgets due to the economic uncertainty brought about by the COVID-19 pandemic, companies are seeking to rid themselves of waste.
Attribution challenges. Buyers are using more channels and devices in their purchase journeys than ever before. The lack of attributive modeling and analytics makes it even more difficult to help them along the way.
Marketers continuing to use traditional channels find this challenge magnified. The advent of digital privacy regulations has also led to the disappearance of third-party cookies, one of marketers’ most useful data sources.
Marketing attribution and predictive analytics platforms can help marketers tackle these challenges. They give professionals more information about their buyers and help them get a better handle on the issue of budget waste.
Read Next: What do marketing attribution and predictive analytics tools do?
Opinions expressed in this article are those of the guest author and not necessarily MarTech. Staff authors are listed here.
Related stories