Automating decisions with real-time situational context
Lisa Loftis from SAS Institute shows marketers at MarTech how they can get value out of data using a decision engine.
“Automating decisions is not a new focus for marketers and CX leaders,” said Lisa Loftis, Principal, Customer Intelligence Solutions at SAS Institute, at our recent MarTech conference. “The issue today is that the pandemic induced digital behaviors that have ratcheted up the importance around automating decisions in CX.”
The expansion of customers’ digital lives has created an explosion of data from increased digital activity across more touchpoints. There is also a greater need for marketers to make decisions in real-time. And this means the process has to be automated, in order to deliver an optimal experience.
“It’s almost impossible for humans to keep up with the sheer volume of customer decisions that have to be made every hour across every department,” Loftis said. These decisions include determining intent, pricing a product or identifying fraud, among countless others.
Data sources
Marketers can gain important insights not just from the data they have, but by identifying where it comes from and basing decisions on that context.
Where does all the data come from? Loftis cited five broad categories.
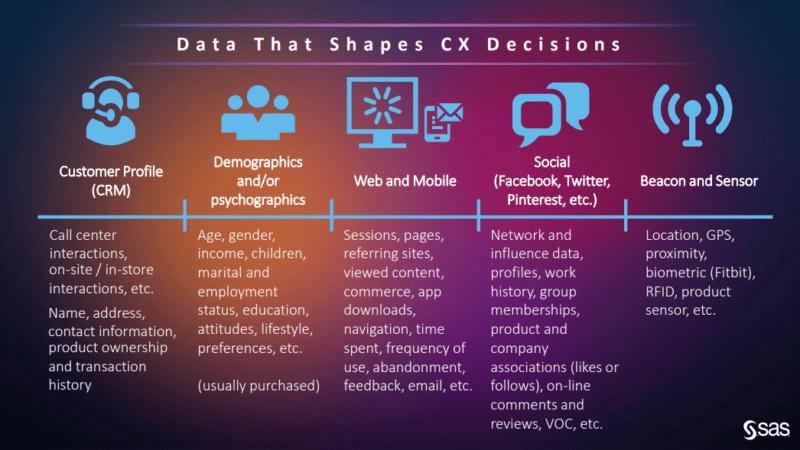
These sources aren’t all of equal importance, but they combine to paint the full picture of a customer, according to Loftis. For instance, many marketers consider call center interactions as the most important source, followed by mobile and web behavior information. Traditional CRM data like purchases and usage info are also near the top of importance for marketers.
Beacon and sensor data out in the world can also rank high because they contribute to making real-time decisions when customers are close to the point of sale, and because the context can help inform these decisions.
“You can map those categories directly into the variety of contextual pointers that companies can glean from their customer information,” said Loftis.
She added, “This process can be incremental in nature, as marketers become more mature in shaping customer journeys and enhancing loyalty with data and analytics.”

Decisioning in action
How do marketers use this data to drive automated decisions? Loftis shared an example from a bank that SAS worked with to implement a decision engine. They were using predictive analytics and automated decisions to deliver optimized product offers in real-time. This engine generated six million leads annually, and over 80,000 new accounts per year. According to Loftis, the bank drove a marketing ROI increase of over 100% within the first few years.
“The engine uses a combination of predictive analytics and machine learning, business rules and predetermined constraints to develop a list of potential offers for each customer,” Loftis explained.
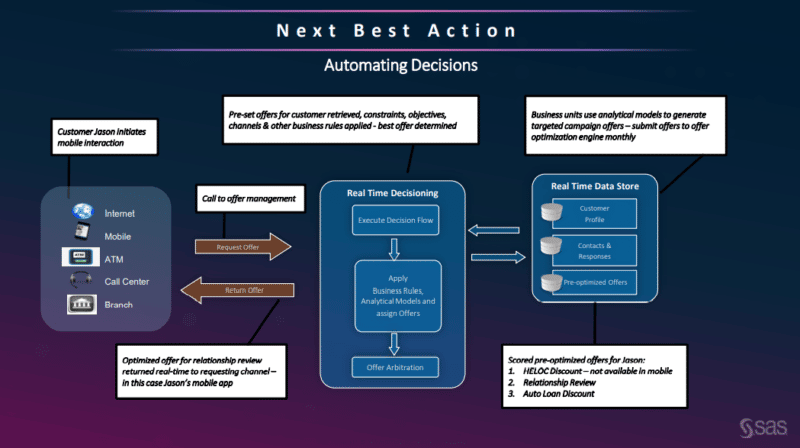
In the above example of one of the bank’s customers, “Jason,” the customer is first engaged on the mobile app or the bank’s website.
“The channel contacts the decision engine for a list of possible offers for Jason,” Loftis said. “The analytically generated and prioritized offers for Jason [in this example] include a home equity line of credit, a relationship review to move him into an account package that better fits his usage patterns and a discounted car loan.”
She added, “The new offer is passed back to the requesting channel, the mobile app, and delivered to Jason, who accepts. This is a win for both Jason, who feels that the bank is looking out for him, and for the bank who has sold a higher-tier product.”
Situational context
Adding real-time situational context to the engine brings another level of relevance to customer engagement and experience.
“It helps to surface those ‘moments of now’ that signal what the customer is doing in a particular moment of time, and it’s what allows marketers to truly meet the customers where they are,” said Loftis.
For example, it’s useful to engage a customer like Jason when he’s at a car dealership. His bank might not know that he’s there, because they aren’t following, and that would be creepy. However, there are contextual clues that will make the bank’s offers to Jason much more relevant.
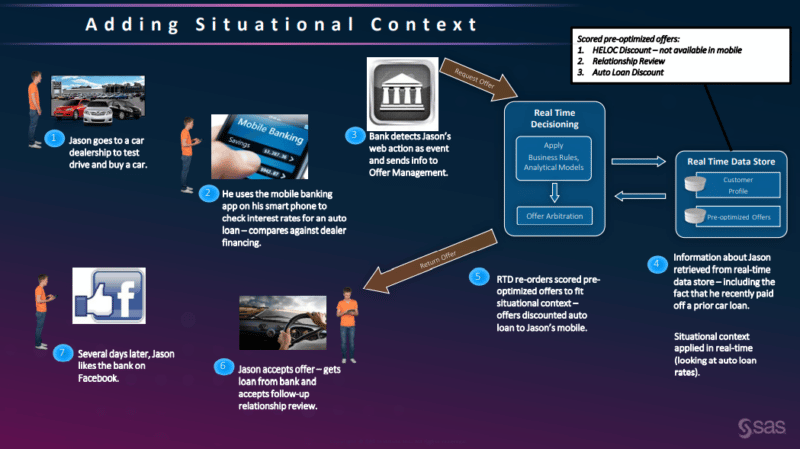
“In this example, Jason is using the mobile app while at a car dealership shopping for a new car,” said Loftis. “Situational context can tell them what he’s doing on the website — he’s looking at auto loan rates. The decision engine is able to combine this real-time web activity with historical transactional behavior from Jason.”
That is, Jason has an existing mortgage with the bank and has also recently paid a car loan off, so he’s already a customer in the CRM. What helps out both the bank and the customer, in this case, is pulling together the relevant data, and doing so in real-time, while Jason is at the dealership.
“The real-time analysis via a scoring model causes the decision engine to re-sequence the offer priorities in a different way than we saw in the prior example,” Loftis said. “So now he’s offered a discounted auto loan rate rather than the relationship review.”
By serving more relevant offers from the decision engine, the customer is happier to hear from the bank. And this means he’s more likely to take the bank up on the offer.
See the full presentation from our MarTech conference here (free registration required).
Related stories